AI coding tools like GitHub Copilot, Cursor, Claude and others are reshaping how software engineers work – offering powerful support across documentation, testing, code comprehension, learning, and more.
For engineering managers, helping their teams embrace and master these tools can lead to significant performance gains, faster onboarding, and stronger collaboration – regardless of seniority. Given how quickly these tools are evolving, it’s essential to start using them now and to continuously train engineers on how to effectively integrate them into their daily workflows.
Let’s explore how these tools can help your team and how engineering managers can guide successful adoption.
4 Ways AI Coding Tools Can Help Engineers
4 Ways AI Coding Tools Can Help Engineers
Engineers are integrating AI coding tools into every stage of the development workflow, from design through to deployment. By coaching teams to adopt these tools you can create a more productive engineering environment and improve the developer experience. Here’s how:
1. Understanding and Gaining Context on Legacy Code
Legacy code can be difficult to understand, especially if an organization lacks institutional knowledge. Fixing bugs and making updates become risky if no one knows how the code works, and one mistake can impact the entire system.
AI coding tools are surprisingly good at parsing unfamiliar areas of code. By breaking blocks of code into logical parts, AI tools help developers identify functionality, understand its purpose, and track dependencies. Once developers have a high-level understanding, they can dig deeper into how legacy code works and refactor with confidence.
I recently came across a regex a coworker of mine wrote. I figured it would take me about 20 minutes to stare at and figure out the regex on my own or 10 minutes to find the co-worker who wrote it and ask them what it meant. I asked Copilot and it instantly decoded the regex for me! I was able to understand what the regex was doing more quickly than I otherwise would with a little help from Copilot.
With tools like Copilot, developers can highlight a section of code and ask the AI assistant to provide natural language descriptions. It’s a quick and easy way to see what a specific piece of code does or how it interacts with other parts of the system. AI coding tools can also spot bugs or bad practices in the highlighted code and suggest improvements.
2. Creating and Maintaining Documentation
Documentation can easily get stale as code bases grow and evolve. Engineers are often working against deadlines, and they don’t always have the bandwidth to keep everything up to date.
Context gaps are a major headache for engineering organizations. Even the clearest code can’t explain reasoning and trade-offs, leaving engineers at risk of making mistakes. Outdated documentation also slows down onboarding – without reliable guides, new team members have to ask a bunch of questions before they can get started.
Most AI coding tools can automatically generate natural language documentation, minimizing the overhead required to keep up with changes. Engineers can ask AI tools to create a README file that introduces and explains the project, or generate inline comments and docstrings. AI also makes documentation more consistent by applying standardized structures, terminology, and tone across the codebase.
Reminder: Your team should review all AI-generated documentation – inaccurate or misleading information could lead to costly errors.
3. Learning New Tech and Languages
Software is evolving rapidly, and building foundational knowledge in new technologies carries a heavy cognitive load. While some organizations promote continuous learning through mentorship, this can be difficult to scale. Mentorship requires time, attention, and expertise, all of which are limited resources.
To help bridge the gap, AI can act as a mentor or guide, showing engineers how to perform a specific task or structure code for the best results. When exploring new frameworks or languages, engineers can ask questions in real time or request examples and templates. AI tools can even review an engineer’s work to catch errors and suggest areas for improvement.
For example, I’m primarily a back end coder. However I sometimes flex into the front end world. I’ve improved over the years so I can easily add Input components or tables and merrily have them send and receive data from endpoints. However, some of the finer points of that last mile pixel pushing do tend to elude me. If our designer asks me to move a label to the side of an input but also slightly closer to the top of the page in order to match some other labels, that sort of thing can end up taking me longer than I would like to admit to figure out. Fortunately, this is the sort of thing that Copilot and its ilk are wonderful at helping with! I can ask over the course of multiple prompts for Copilot to first move the label to the side and then adjust it to be inline with the other labels. If I don’t understand the code, I can even ask Copilot to explain it back to me! I’m still learning, just faster.
For engineering teams growing at scale, AI mentorship is a valuable addition. In contrast to their human counterparts, AI mentors are always available, easy to scale, and able to provide consistent guidance without burnout.
4. Generating Tests
Rapid software development sometimes comes at the cost of high-quality code. Engineers with tight deadlines are more likely to skip or rush testing, which can let bugs slip through and make code more difficult to maintain.
AI coding tools help engineers maintain high testing coverage while focusing their time on development. By analyzing the code’s structure and logic, AI can automatically generate unit tests to validate expected behavior and identify edge cases. Developers can also use AI to target untested sections of code to generate additional tests that improve coverage and reliability.
You can use Copilot editor inline chat /tests command to select functionality and almost magically create a whole test suite for your code. If you are looking for something more specific, my colleague Daniel recently used Copilot chat to describe over the course of several prompts the type of test cases he was looking for and the type of input data he wanted. Copilot provided him with a basis to start from and from there he was able to update and add more detail and additional test cases.
Or perhaps like my colleague Jesse you want to write tests in a specific syntax, such as Lint rules. AI helps Jesse write these tests, since writing Lint tests isn’t part of his daily life. AI can help him dust the cobwebs off and remember how to achieve the functionality he is looking for.
Sometimes AI can act like a well-informed co-worker to bounce ideas off. My colleague Nic recently was thinking about data fidelity tests and how to optimize the SQL for these. He found that by asking an AI Model for thoughts as he was working on these over the course of a day he was able to get where he wanted to be at rocket speed!
Manual testing still matters, especially for critical features like payment systems. Using AI tools to move faster and quickly create boilerplate ensures developers save manual testing for complex, high-impact areas.
Mastering the Art of Prompting
Mastering the Art of Prompting
To get the best return on investment for your AI coding tools, engineers need to become skilled at prompting. Engineering leaders should discuss best practices with their teams and encourage experimentation with different prompts – it’s the best way to figure out what works and what doesn’t.
Remind your engineering team to take it a step at a time when learning to prompt. Start with out-of-the-box commands and use the chat functionality to refine the result. With each attempt, they’ll reach the desired outcome faster and with fewer, more precise prompts.
Formulating the right question to ask an AI tool isn’t always easy – subtle differences in the wording, tone, or format of a prompt often yield distinct results. Testing different versions of the same question helps engineers fine-tune their engineering prompts and gain control over the outcome.
Advise your team to give clear, detailed instructions so that the AI coding tool knows exactly what they want to achieve. A good coding prompt typically goes beyond what you want the code to do, specifying the programming language, input and output formats, and edge cases.
Tackling Barriers to Adoption
Tackling Barriers to Adoption
“You don’t trust me to write my own code.”
“You want robots to replace us.”
“You’re forcing me to use these tools.”
“You’re going to measure my performance based on this.”
Not everyone in your organization will be excited about AI coding tools. Some engineers may push back against AI, and you’ll need to anticipate and address their concerns to achieve widespread adoption.
Encourage engineering teams to view AI coding tools as a means to increase their impact rather than a potential threat. You can reduce fears by introducing AI coding tools to a small group of internal advocates who can evangelize its use and best practices. Be sure to share and celebrate their success stories with the rest of the organization.
Consider pairing engineers who need more support with AI adoption with an advocate who can help them work through the challenges and achieve easy wins early on. It’s also important that everyone on the team understands they can still ask their colleagues for help – AI coding tools support rather than replace collaboration and mentorship.
Last but not least, be patient and expect a fair amount of ramp time. Everyone learns at a different pace, and it’ll take time to achieve organization-wide proficiency. Focus on creating a community that shares best practices, successes, and failures as they move through the learning process.
AI coding tools have the potential to level up every engineering organization. With the right approach to coaching and adoption, you can future-proof your workforce and build more motivated, more productive engineering teams.
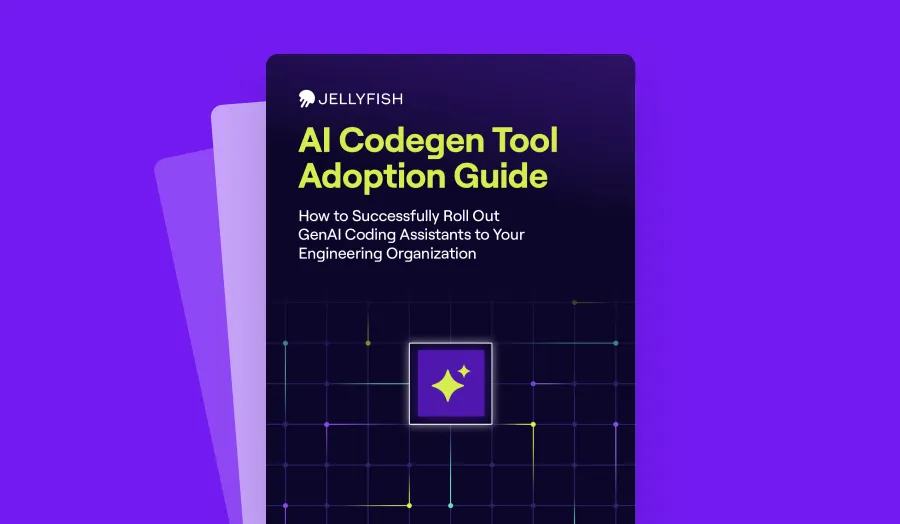
AI Coding Tool Adoption Guide
Ready to get started with AI coding tools? Jellyfish can help.
About the author
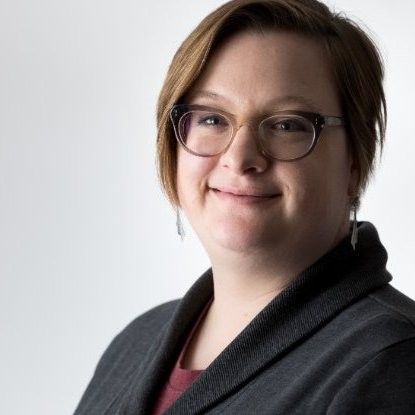
Greta is a Backend Engineer at Jellyfish. She has experience in Python, Node.Js, React, SQL, MongoDB, Typescript, and PHP and has worked with AWS Lambdas, Django, GraphQL, Sentry and Datadog.